Hong Kong Data Science for Companies
Why do we need data scientists?
In essence, data scientist makes any business grow better, be it finance, health care, transportation, or telecommunication. They empower businesses to make better decisions with quantitative insights. They challenge preconceived ideas, identify opportunities, test hypothesis of different decisions and more.
In the age of AI, data scientists leverage machine learning tools to process big data. The technical knowledge that they bring to the table allows is crucial to process and analyze data more efficiently. Data scientist also have the needed business acumen to discover business insights accurately and make their findings relevant to the organization.
What is data science?
Data science is the field of study that combines domain expertise, programming skills, and knowledge of math and statistics to extract meaningful insights from data.
Data science practitioners apply machine learning algorithms to numbers, text, images, video, audio, and more to produce artificial intelligence (AI) systems that perform tasks which ordinarily require human intelligence.
In turn, these systems generate insights that analysts and business users translate into tangible business value.
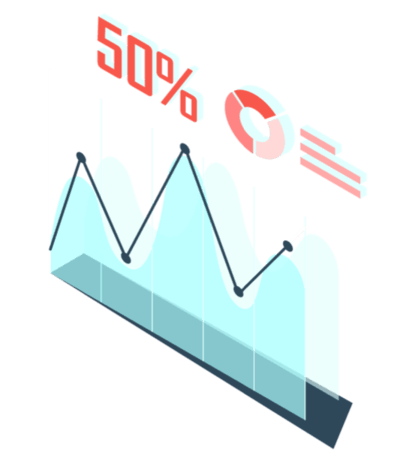
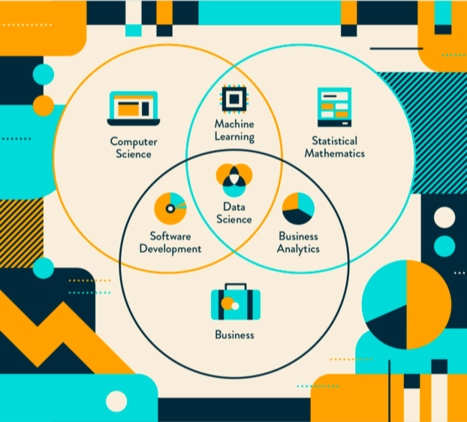
What are data scientists?
Data scientists have to understand math and statistics, programming and database, domain knowledge and soft skills, and communication and visualization. With these diverse skillsets, they can transform data into insights. Their job requires them to not only know how to process and leverage the vast amount of data they have at hand, but also communicating their findings with their team and presenting them to clients.
Recently, data scientist has been a very hot job and was even named as “the sexiest job of the 21st century”. According to Indeed.com, job postings for data scientists quadrupled in 5 years’ time since 2013. The convergence of data and business and the increasing importance of data in today’s society have increased the hype and need for data scientists. More and more corporations find it essential to truly understand what data means to the company and its underlying potentials. In hindsight, the role of data scientist appeals to many prospective students and job seekers due to its job diversity and high pay.
What are some data science solutions that our company can easily implement?
Each company’s pain point is different. Corporations may have the luxury of having a large amount of data, yet, in a lot of cases those data may not be clean. On the other hand, smaller companies’ data may be clean but do not have a lot of data they can play with.
Understanding that not all companies’ data is ready or sufficient for creating data solutions, ThinkCol has helped some of its clients to create solutions involving Natural Language Processing (NLP) and computer vision. Some NLP solutions includes sentiment analysis and information extraction while computer vision solutions involve people counting and monitoring customer behavior. We are able to leverage data from NLP and computer vision solutions.
Aside from this, some common data solutions that we have worked with clients are: data visualization and data cleaning. Data visualization is an easy way for us to visualize the data at hand, uncover any interesting insights and understand whether there are faulty items. Contact us at info@thinkcol.com, so that we can make your data dreams come true!
Why does data science matter to me or the company if I’m not in the field of analytics?
According to KPMG, 80% of enterprises rely on analytics to improve their understanding towards their customers. With huge competition in most markets due to globalization, analytics is transformative to any line of business, not only because it can help companies to increase profit, but also to understand competition and conduct research.
With employees that showcase these traits, companies can easily incorporate data science and AI practices and use data science to guide business decisions. This allows digital transformation to happen through a top-down approach and a bottom-up approach.
It’s important for everyone in the company to have the mindset of a data scientist. Traits include being comfortable with using data, understand basic data and AI concepts, being curious, having strong business acumen and willing to communicate and collaborate with people.
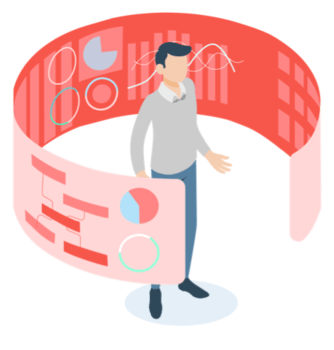
I’m not a data scientist, but would like to be one. What should I do?
There are also more and more masters programs about data science in Hong Kong. For instance, HKU’s Master of Data Science or Master of Science in Business Analytics, CUHK’s M.Sc. in Data Science and Business Statistics and HKUST’s Master of Science in Big Data Technology.
If you are a working professional, there are also various data science courses to take online and offline. There are various online materials available in Coursera and Kaggle.
Data science short courses and high diplomas for different concentrations are available in HKU Space. Our co-founder, Kane Wu, also teaches courses in HKU Space for working professionals. Subjects like big data and machine learning, its applications, Cloud computing and data science are covered in various classes. There are also online materials available in Coursera and Kaggle. Coursera provides courses from well-known universities. One of which is provided by John Hopkins University on a Data Science Specialization, covering 10 courses from R Programming to Machine Learning.
Kaggle, on the other hand, is an online community of data scientists and machine learning practitioners. It is a public data platform and also offers machine learning competitions.
Microsoft also have resources and its own Data Scientist certification. By taking their DP-100 exam, you can be a Microsoft Certified: Azure Data Scientist Associate. Chapter by chapter online materials for the course can be easily accessed on Microsoft’s website. You can also gain first hand experience in understanding how Azure, a cloud computing service, works.
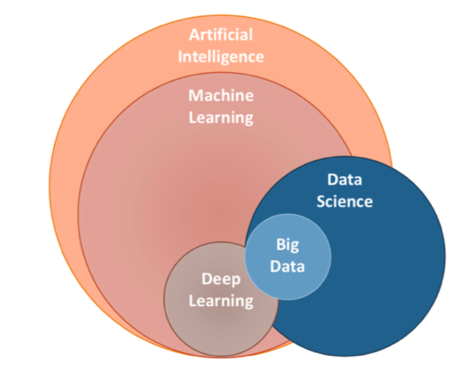
I’m interested in knowing more about data science. What are some data science groups I can join in Hong Kong?
HK Data Science Society hosts regular events about data science and have a community of data scientists within the society!
Is AI and data science related? How do they converge?
Even though data science overlaps with AI in many areas, data science and artificial intelligence are not exactly the same. Data science applies AI and other mathematical methods to discover insights and also includes data visualization and data science consulting. Data science also uses data science consulting and data visualization to find business values for different corporations
On the other hand, AI is the ability of a digital computer or computer-controlled robot to perform tasks commonly associated with intelligent beings.
Does ThinkCol create data solutions?
Yes we do! As an AI consultancy firm, data science is an indispensable part of ThinkCol’s work. Over the years, we have created dashboards, cleaned data, labelled data, led data exploration and data science projects for multinational corporations, industry powerhouses and governmental organizations.
ThinkCol has our own team of data scientists, project managers, UI/UX designers who have continuously helped myriad clients achieve their data and AI goals. We are capable in handling data solutions and would love to work with you. Contact us at info@thinkcol.com to get in touch! We’ll get back to you as soon as possible.
What if I don’t know how data science can help my organization?
We have consulted a lot of organizations that experience the same pain point. Whether it’s an industry powerhouses or a company of smaller scale, myriad companies face the same problem. Having worked with numerous organizations that range of different industries, we curated a set of tried and tested curriculum and methodology that works for our clients.
AI idea generation is a crucial element of our training. We prepare participants for brainstorming AI ideas through showcasing relevant AI technology, use cases, and design thinking. We’ve found that with our framework and our worksheets, participants can think of AI solutions that solve actual pain points of participants.
Our framework also helps with the implementation front, such as whether the client’s data is ready to implement AI solution, risk and privacy concerns as well as budgetary estimation. We encourage participants to think of solutions to potential data and implementation challenges, so that our client can identify some champions to lead future AI projects during the workshop.
ThinkCol also delivers a customized AI Roadmap Report for ideas after the workshop, so that you can kickstart your digital transformation journey through the ideas generated in the workshop.
In the past, we’ve worked with multiple industry powerhouses across various industries – ports, logistics, retail, manufacturing, jewelry as well as quasi-governmental organizations on delivering 8 to 13 weeks as well as one day AI and data science workshop.
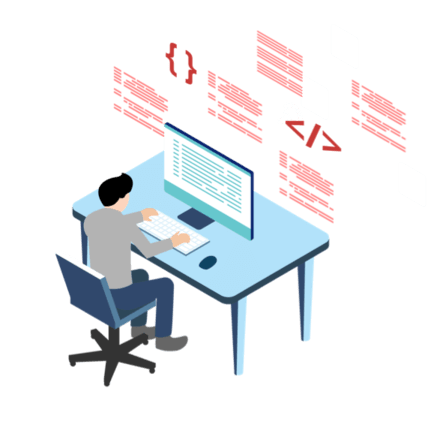
How does ThinkCol differ from other companies when approaching data science projects?
We believe that data science should always be customer centric. The ThinkCol process puts customers’ needs as the vocal attention and is aimed at delivering fast gains. We pride on solving customer pain points as their AI partner.
ConsultationAligning expectations and defining accuracy
We understand that if we do not engage our clients and business users periodically, it will be unlikely that our solution is adopted. It is only when all parties understand the technology involved and align on common goals can the data or AI solution be useful to a business.
We conduct in-depth consultation and have meetings continuously during our projects, so as to ensure the right product is provided to the users and that we fully understand the customers or the business users’ needs and pain points.
We also explain machine learning and data science in a laymen language, so that the client would have faith in the product we create. With the client and business users, we define the project’s expected accuracy and methods to test the solution so as to ensure it works during the implementation.
Our consultations may not only include management, it may also engage various stakeholders to allow us get a grasp of the full work flow and expectations. In a lot of cases, management level may not understand the problem to the full extent as the day-to-day work are done by their subordinates.
Review and analyze sample data
After the initial consultation, we conduct further data understanding. This may include understanding the data that the organization has and doesn’t have, exploring other methods of acquiring data if there isn’t sufficient data available, inspecting the cleanliness of the client’s data, pointing out any data challenges that may affect the project, reviewing how data is stored etc. During this process, we usually request some sample data from the client.
In fact, a lot of our clients ask us what we can create using certain data that they have. We not only have to understand what data they have, but also which departments use it, how they use it, what are the business processes, how clean is the data and what are the business applications etc.
In many cases, we are not only just dealing with one data source from a single department, the data available may be cross-departmental and usually, quite messy. After understanding the business framework and essential data questions, ThinkCol comes up with appropriate business applications that are essential to the client and works with them to make it a reality.
Project ScopingExplore hybrid solutions and prioritize objectives
Before we sign any contract with the client, we explore various possibilities in tackling the same problem, so that the client has the flexibility in choosing a solution that suits their budget. We would also layout the timeline and resources needed. Project scoping is important for both the client and to us, as a partner or a vendor, as we can align on expectations and understand how to work with each other.
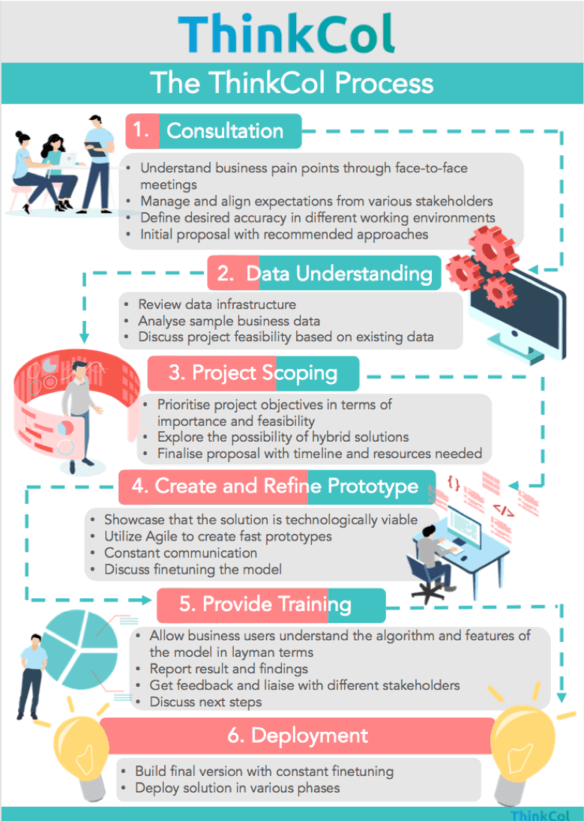
Showcase viability of solution
We usually advice clients to engage in a Proof of Concept (PoC) before committing to the adoption of any new technologies or processes. A PoC demonstrates our capability in the specified technology. The PoC would usually last for less than 2-3 months, so as to showcase the solution is technologically viable. We would communicate with our client regularly, so as to ensure the specifications are met and challenges are raised accordingly. We would also finetune the solution according to the clients’ feedback.
For instance, a retailer wanted us to create a tool so that they could visualize their human resources. As a PoC, we first helped them to create a tool that only focused on their business in Hong Kong. After the PoC, they were happy with the tool and we continued to help them with visualizing their data for all of the regions that they had businesses in.
For one of our clients, Lenovo, we deployed more than 100 models to automatically tag and summarize, understand comments from different continents (Spanish, Portuguese, English). However, before we included all of the languages and regions, the project started as a small Proof of Concept. In the PoC, we only built models to understand English comments. After a successful PoC, we progressed building models for other languages and more products. We even created a user interface that allowed Lenovo users to build their own models and empower Lenovo staff to maintain the model themselves. By doing so, we created a self-sustaining solution for the user.
Provide TrainingGet feedback
We put particular emphasis in training users in utilizing the new solution that we’ve created because we understand that without proper training, the new solution would not be adopted. At the same time, we also impart knowledge about data science and AI to users to ease communications and understand how the model can be improved.
DeploymentWith the feedback of various stakeholders’, we finetune the model accordingly and build the final version of the model. This may involve further planning with different business users as the new system may affect business processes.
ThinkCol believes in producing self sustaining solutions. After we create the solution, we help to train users and also help with recruitment so that the client can maintain the solution by themselves.
What are some AI and data science terms that are useful to know?
Machine Learning - An application of AI that provides systems the ability to learn automatically and improve from experience without being explicitly programmed.Supervised Learning – A Machine Learning method in which we teach the machine using labelled data.
Unsupervised Learning – A Machine Learning method in which the machine is trained on unlabeled data without any guidance.
Deep Learning – An AI function that mimics the workings of the human brain in processing data for use in detecting objects, recognizing speech, translating languages, and making decisions.
Natural Language Processing (NLP) - A branch of artificial intelligence that helps computers understand, interpret and manipulate human language. Machine translation and sentiment analysis utilizes NLP.
Internet of Things (IoT) – The interconnection via the Internet of computing devices embedded in everyday objects, enabling them to send and receive data.
Computer Vision - A field of computer science that works on enabling computers to see, identify and process images in the same way that human vision does, and then provide appropriate output.
Predictive Analytics - A category of data analytics aimed at making predictions about future outcomes based on historical data and analytics techniques
Propensity Modelling – Used to predict the behaviour of customers.
Segmentation – By splitting customers into groups based on their profiles and past behaviors, we can identify customer groups to target.
Churn prediction – Utilizing statistical modelling to predict the likelihood a customer switches to a competitor product. By knowing such information, we can generate more incentive, such as certain discounts, for those particular customers.
Forecasting – Using past data to identify a pattern and project out into the future. For instance, forecasting the demand of a certain product based on past transactional data.