Navigating the Future: Understanding Agentic AI, AI Agents and Its Potential for Hong Kong
As artificial intelligence continues to evolve at a rapid pace, businesses and organizations are increasingly looking for ways to leverage these technologies to drive innovation and efficiency. Among the emerging paradigms in AI, agentic AI stands out as a powerful approach that goes beyond simple content generation. At ThinkCol, Hong Kong's leading AI consultancy, we're at the forefront of implementing these advanced AI systems for businesses across various sectors. This aims to clarify the distinctions between different AI approaches, explain how agentic AI is implemented, explore its use cases, discuss ThinkCol's implementations in Hong Kong, address potential drawbacks, and examine the relationship between MCP and agentic AI.
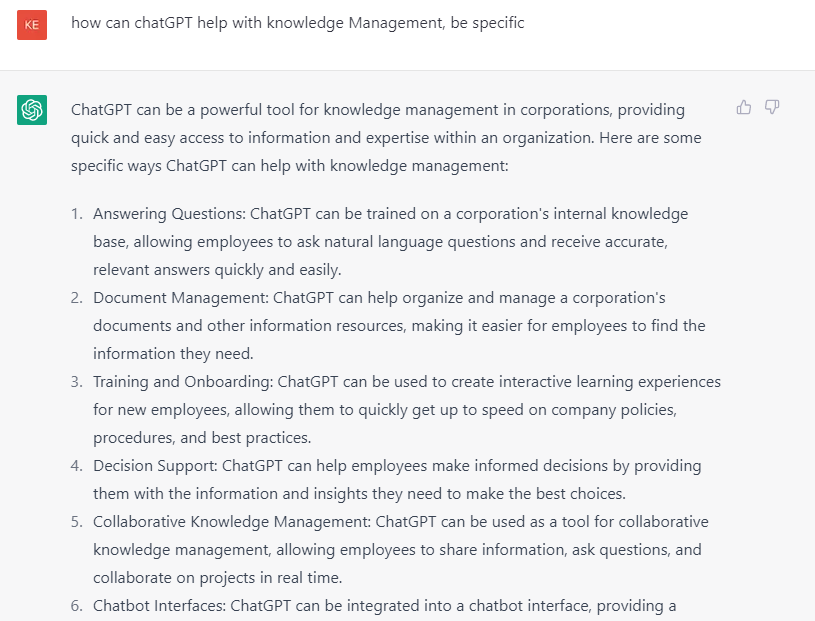
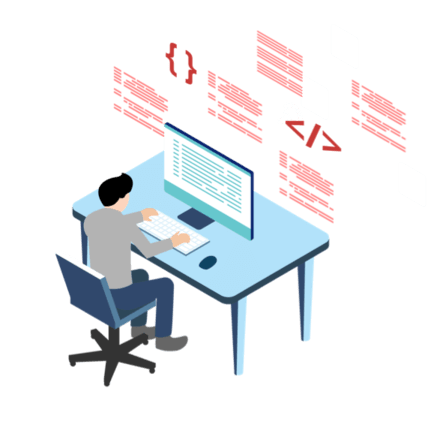
Understanding the AI Landscape: Generative AI, AI Agents and Agentic AI
The AI field is filled with numerous terminology that can sometimes overlap or cause confusion. Let's clarify the key differences between three important concepts: generative AI, AI agents, and agentic AI.
Generative AI: The Creative Force
Generative AI refers to artificial intelligence systems designed to create original content based on patterns learned from vast amounts of training data. These systems can produce text, images, video, audio, or software code in response to user prompts. Generative AI waits for a prompt and then generates content based on that input. Examples include ChatGPT, DALL-E, and MidJourney, which have revolutionized content creation across various industries.
AI Agents: The Individual Performers
An AI agent is theoretically a single entity designed to handle multiple tools and tasks with one prompt. It operates autonomously to achieve specific goals with limited supervision. Unlike traditional AI models, which operate within predefined constraints, AI agents exhibit autonomy, goal-driven behavior, and adaptability to changing environments. These agents can make decisions and take actions based on their understanding of tasks and contexts.
Agentic AI: The Collaborative Orchestra
Agentic AI takes the concept of AI agents further by coordinating multiple specialized agents to work together toward a common goal. This approach involves different types of agents – such as SQL agents, analysis agents, and file generation agents – each with their own specialization, working under the coordination of a planning agent that orchestrates their efforts. Agentic AI systems don't just generate outputs; they make decisions, take actions, and adapt to changing environments in pursuit of specific objectives.
The fundamental difference lies in their purpose and autonomy: generative AI creates content, while agentic AI accomplishes tasks through decision-making and autonomous action with minimal human input.
Implementing Agentic AI: Tools and Frameworks
Implementing agentic AI requires specialized frameworks and tools that enable the orchestration of multiple AI agents. Here are some of the most prominent approaches:
AutoGen: Orchestrating Agent Conversations
AutoGen is a powerful framework for orchestrating AI agents, making it easy to build modular AI workflows where each agent handles a specific task. It offers a unified multi-agent conversation framework as a high-level abstraction for using foundation models. AutoGen provides mechanisms for agent communication protocols, conversation patterns, and integration with external resources, allowing developers to create complex, collaborative problem-solving systems.
LangGraph: Structuring Multi-Agent Workflows
LangGraph provides a framework for building multi-agent workflows, allowing developers to structure how different agents connect and interact. It enables creating networks of agents that can collaborate on complex tasks, with different patterns for collaboration including shared scratchpads, agent supervisors, and hierarchical agent teams.
Model Context Protocol (MCP): Bridging Data Sources
MCP is an open standard that allows AI models to access files, APIs, and tools directly without intermediate processes like embeddings or vector searches. An MCP server acts as a bridge between AI models and various data sources, enabling real-time query and retrieval of information. This protocol enables agentic AI systems to access the data they need efficiently, enhancing their ability to make informed decisions and take appropriate actions.
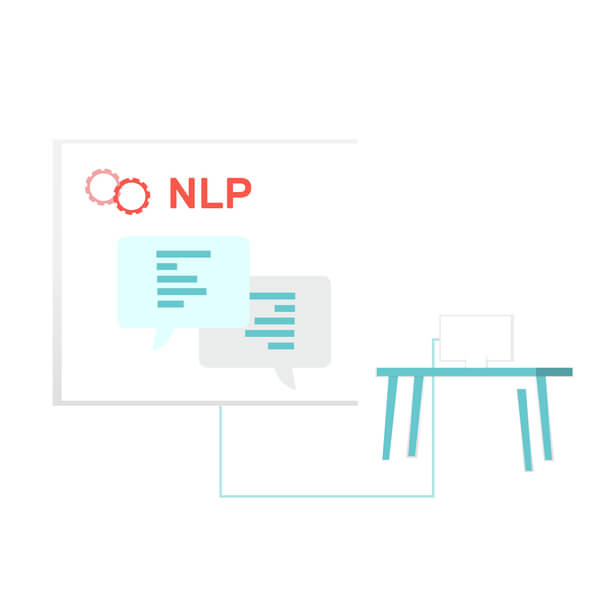
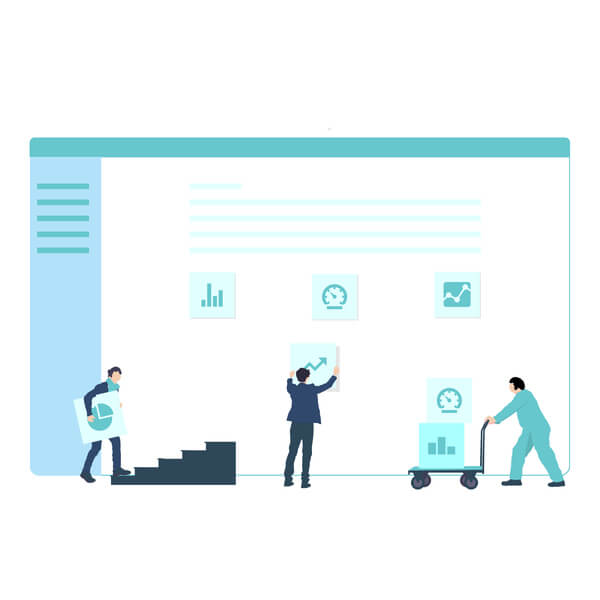
Agentic AI Use Cases: Transforming Industries
Agentic AI is finding applications across various industries, transforming how businesses operate and solve complex problems:
Software Development
AI agents can function as developers, writing, testing, and debugging code autonomously. They can break down complex programming tasks into manageable components, work through technical challenges, and even optimize existing codebases for better performance.
Knowledge Management and Research
As research assistants, agentic AI systems can access multiple data sources, analyze complex information, generate insights, and create comprehensive reports. They can manage information across different databases and formats, making them valuable tools for knowledge-intensive industries.
Business Process Automation
In sectors like insurance, human resources, retail, and e-commerce, agentic AI can automate complex workflows that involve multiple steps and decision points. For instance, in insurance processing, different agents can handle document analysis, risk assessment, policy comparison, and customer communication, all coordinated by a central planning agent.
Supply Chain Optimization
Agentic AI can transform supply chain management by coordinating different aspects such as inventory management, demand forecasting, logistics planning, and supplier communication. By having specialized agents for each of these functions working together, businesses can achieve more efficient and responsive supply chains.
The Challenges of Agentic AI: Understanding the Limitations
While agentic AI offers tremendous potential, it's important to understand its limitations and challenges:
Operational Complexity
Implementing agentic AI often involves long running times and numerous system calls. The complexity of coordinating multiple specialized agents can lead to over-engineering, particularly when simpler solutions might suffice. In many cases, businesses don't actually need a complex multi-agent system to solve their problems effectively.
Maintenance Nightmares
Maintaining AI agents presents significant challenges. As highlighted by industry experts, up to 95% of automation work after initial creation lies in maintaining the processes. The current agentic models offer limited solutions for ongoing management and adaptation, making long-term sustainability a concern.
Reliability and Error Rates
AI systems are fundamentally imperfect. Even with high accuracy rates, large enterprises could face hundreds of errors monthly, each potentially leading to significant financial losses or reputational damage. This inherent unreliability makes agentic AI solutions risky in environments where precision is paramount.
Governance and Control
The rise of citizen development in AI poses significant risks to enterprise governance. Without proper oversight, employees across an organization could create their own AI agents, leading to a chaotic landscape of uncontrolled automation without clear processes. This "shadow AI" challenge requires careful management and clear governance frameworks.
Data Dependency and Bias Risks
Agentic AI's effectiveness depends on high-quality, diverse, and up-to-date data. Any deficiencies in the data pipeline can manifest as bias, inaccuracies, or errors in decision-making. Bias in training data can lead to automation systems producing suboptimal or unfair results, particularly in customer-facing applications.
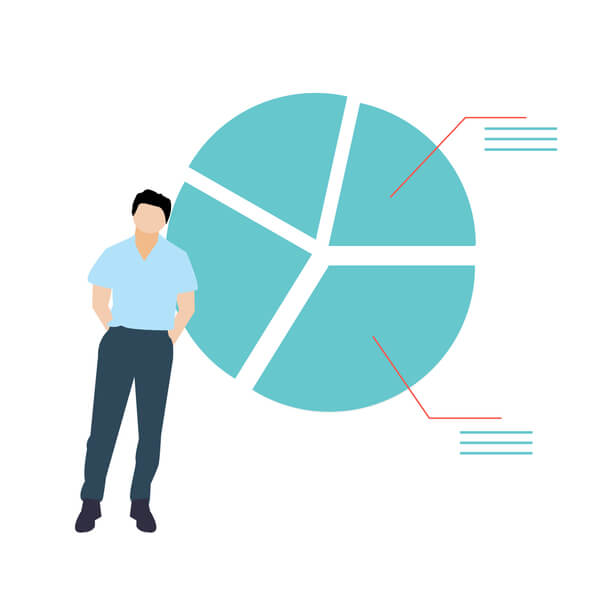
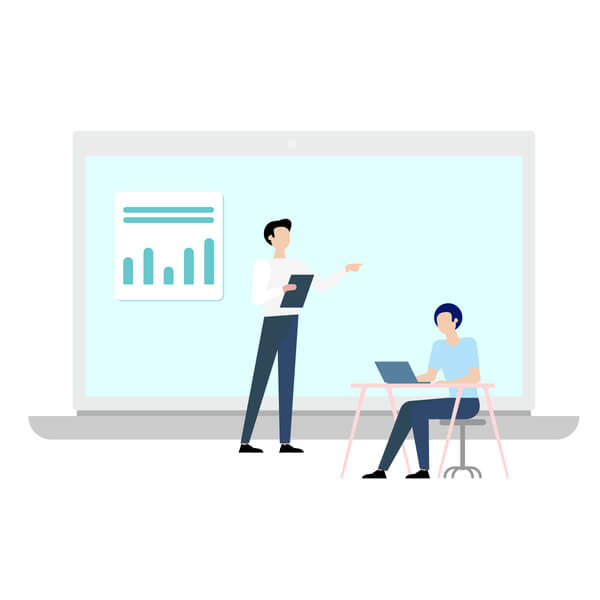
How can ThinkCol Help with Agentic AI
Agentic AI offers remarkable opportunities for automation, efficiency, and business transformation, but it also introduces unique challenges-including model reliability, data quality, system complexity, and the need for specialized talent. ThinkCol, as a leading AI consultancy in Hong Kong, is uniquely positioned to help organizations navigate and overcome these obstacles.
1. Customized, End-to-End AI Solutions
ThinkCol specializes in designing and deploying tailored AI and GenAI solutions that address specific business pain points. By working closely with clients to understand their unique requirements, ThinkCol ensures that agentic AI systems are not just technically sound but also aligned with real-world business goals. This approach helps prevent over-engineering and ensures that AI initiatives deliver tangible value.
2. Enhancing Model Reliability and Predictability
Agentic AI systems can sometimes produce unpredictable results due to their autonomy. ThinkCol addresses this by implementing robust validation, monitoring, and feedback mechanisms within its solutions. Features such as performance monitoring, alerting for irregularities, and continuous validation ensure that agentic AI outputs remain accurate, relevant, and aligned with organizational objectives. This reduces operational risks and builds trust in automated decision-making.
3. Ensuring High-Quality, Real-Time Data
For agentic AI to perform optimally, it needs access to accurate and timely data. ThinkCol's expertise in ETL, cleansing, and integration ensures that AI agents draw from high-quality data pipelines. The company's platforms support advanced data processing and retrieval methods, enabling agentic systems to make informed decisions based on the latest and most relevant information.
4. Streamlining Deployment and Maintenance
Agentic AI can be complex to deploy and maintain. ThinkCol's processes is designed to facilitate automatic deployment, version control, and chatbot orchestration, making it easier for enterprises to move from proof-of-concept to production at scale. This reduces the technical burden on in-house teams and accelerates time-to-value.
5. Building AI Capability and Talent
Recognizing that successful agentic AI adoption requires skilled talent, ThinkCol provides data science training, workshops, and hackathons to upskill client teams and foster a culture of innovation. This helps organizations build the internal expertise needed to manage and evolve their AI systems over time.
6. Robust Governance and Security
With increasing concerns around data privacy and system governance, ThinkCol incorporates best practices in AI governance, ensuring that agentic AI deployments comply with regulatory standards and organizational policies. This minimizes risks related to data security and uncontrolled automation.
Conclusion
Agentic AI represents a significant evolution in artificial intelligence, moving beyond content generation to autonomous decision-making and action. By coordinating multiple specialized agents, agentic AI systems can tackle complex tasks that would be challenging for individual AI models. At ThinkCol, we're leveraging frameworks like AutoGen, LangGraph, and MCP to implement cutting-edge agentic AI solutions for businesses in Hong Kong.
While the potential of agentic AI is immense, it's important to approach its implementation with a clear understanding of its limitations and challenges. Not every problem requires a complex multi-agent system, and the maintenance, reliability, and governance challenges associated with agentic AI need careful consideration.
As AI continues to evolve, ThinkCol remains committed to helping businesses navigate this complex landscape, implementing solutions that drive real value while managing the associated risks and challenges. By staying at the forefront of AI innovation, we're helping Hong Kong businesses prepare for a future where intelligent, autonomous systems play an increasingly important role in business success.
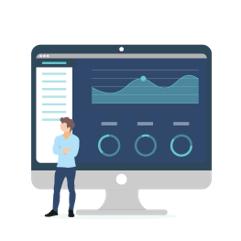